PREDICTIVE MAINTENANCE
- Predictive maintenance entails monitoring the condition of essential machinery to track performance, and to detect possible defects that could result in a system crash.
- The global predictive maintenance market is expected to grow to$6.3 billion by 2022, according to a report by Market Research Future.
According to a PWC report, predictive maintenance in factories could:
- Reduce cost by 45 %
- Improve uptime by 60 %
- Reduce safety, health, environment, and quality risks by 52 %
- Extend the lifetime of an aging asset by 46%
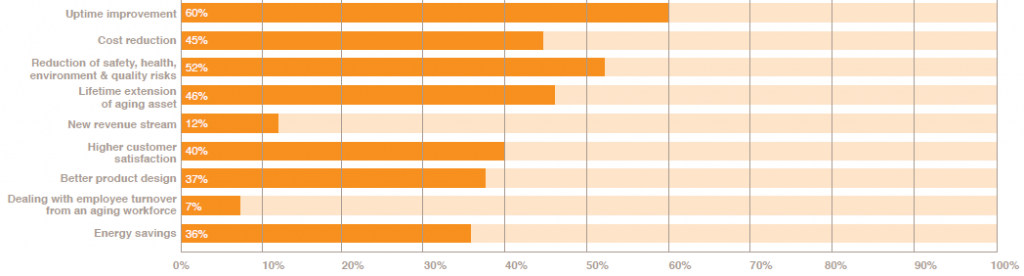
How Much Is Equipment Downtime Costing Your Workplace?
- Unplanned downtime results in loss of customer trust and productivity: 45 % couldn’t deliver services to customers, 37% lost production time on a critical asset, and 32% were totally unable to service or support specific equipment or assets.
- The five industries most impacted by equipment downtime in terms of revenue lost per hour are pharmaceuticals ($2.1 million);insurance ($2.5 million); information technology ($3.3 million); telecommunications ($4.6 million); and financial services ($8.2 million).
Predicting Engines Failure for Airlines
Airlines are interested in predicting engines failures in advance to enhance operations and reduce flight delays.
Observing engine’s condition through their sensors and that’s will facilitate this type of maintenance by predicting Time-To-Failure (TTF) of in-service engine.
Maintenance work could be planned according to TTF predictions instead of complement costly time-based preventive maintenance.
By Using Expected Value method to calculate business gain in monetary units, we were able to support decision-making in different operating conditions.
The data provided is simulated aircraft engine( 20,000 cycle records for 100 engines.) run-to-failure events, operational settings, and 21 sensors measurements are provided by Microsoft.
Predicting the engines’ Failure in the Future based on historical failures of aircraft engine’s sensor values over time.
Learning the relationship between sensor values and changes in sensor values.
Machine Learning Algorithms will be implemented to predict the below:
- Engine Time-To-Failure (TTF).
- Features which have high impact on Engine Time-To-Failure(TTF)
- Expected Profit per engine at the optimum working capacity.
Our Model answers the following Questions:
What are the Features which have high correlation with Time-To-Failure(TTF)?
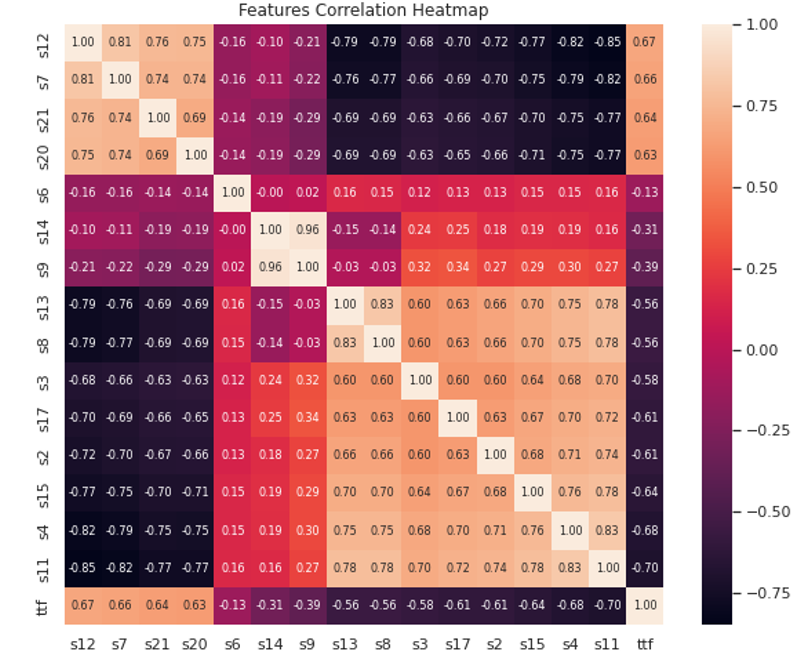
As seen in the Correlation Heat map that 10 sensors out of 21 sensors:
‘s12’, ‘s7’, ‘s21’, ‘s20’, ‘s3‘, ‘s17’, ‘s2’, ‘s15’, ‘s4’, ‘s11‘
Have high correlation with the Time-To-Failure(TTF)
What is the Prediction Time-To-Failure(TTF)?
- The Model Predict the TTF for each engine.
- The Model predicts TTF within average error range of ±28 cycles
What is Expected Profit per engine at the optimum working capacity?
Our Model prediction will produce expected profit of $19K per engine If there is a maintenance capacity to serve %31 engines per period, as per below assumptions:
- Engines need maintenance and selected by the model, has benefit of $300K
- Engines that are fine and not selected by the model, has benefit of $0K
- Engines that are fine but selected by the model, has cost of $-100K
- Engines need maintenance but not selected by the model, has cost of $-200K